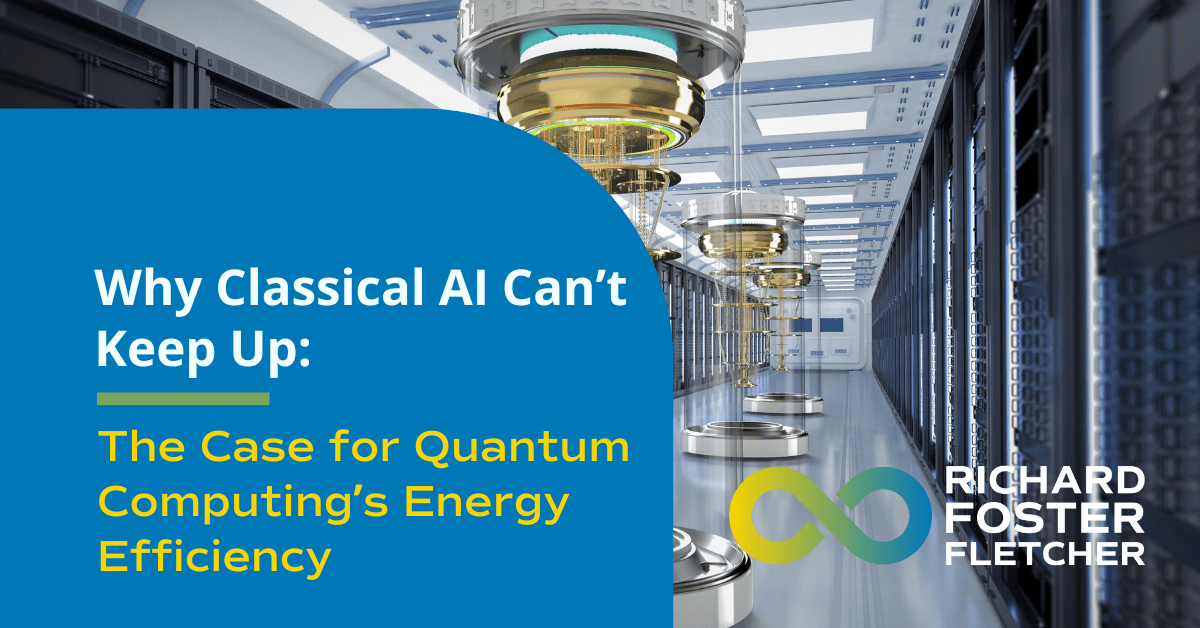
Why Classical AI Can’t Keep Up: The Case for Quantum Computing’s Energy Efficiency

As artificial intelligence scales up, so do its power demands, posing serious challenges for sustainable growth. Training massive AI models on traditional computing systems, even the most advanced GPUs, is becoming costly in terms of both energy and infrastructure. Peter Chapman, CEO of quantum computing company IonQ, likens this reliance on classical systems to “trying to hammer a nail with a banana.” In other words, we’re using the wrong tool for the job – an approach that’s neither efficient nor practical as AI models continue to grow in complexity.
This analogy highlights a critical issue: classical computing simply isn’t equipped to handle the rising energy and cost requirements of advanced AI. Quantum computing, by contrast, presents a solution. With its unique approach to processing data, quantum offers a way to dramatically cut energy consumption and costs, potentially transforming the AI landscape.
The Limits of Classical Computing for AI
Today’s AI models require billions, even trillions, of calculations to train effectively. Data centres packed with GPUs currently handle these tasks, but they do so at tremendous cost in both energy and money. While companies are investing in massive data centres and even exploring alternative energy sources like nuclear power to keep up with demand, these solutions are reactive rather than addressing the core issue: classical computing architectures were not designed for the high-complexity workloads that modern AI demands.
As Chapman’s analogy suggests, even the most powerful classical systems become inefficient when applied to tasks that exceed their intended design. Traditional CPUs and GPUs handle linear, sequential processing well, but they struggle with the parallel, probabilistic calculations that many AI models require. This mismatch in capability leads to rising energy needs and costs that will only become more prohibitive as AI models continue to expand.
Why Quantum Computing is Better Suited for AI
Quantum computers, by contrast, are inherently suited to handle the kinds of complex calculations that AI requires, and they can do so with far greater efficiency. Classical computers process data in bits, representing either a 0 or a 1. Quantum computers, however, operate with qubits, which can exist in multiple states simultaneously. This feature allows quantum systems to process exponentially more information at once, making them far more energy-efficient for certain types of calculations.
For AI, this capability is transformative. Quantum computing’s architecture allows it to evaluate a vast array of possibilities simultaneously, making it ideal for AI tasks that involve extensive pattern recognition, large-scale optimisation, or complex simulations. For example, training a language model on a quantum processor would require significantly less energy and time than it would on a traditional GPU. The computational efficiency of quantum technology could allow us to run AI models that are more powerful and sophisticated without driving up costs and energy demands.
The Search for Quantum’s “Killer Application”
In the 1980s, personal computers found their first “killer application” with the invention of the spreadsheet, which demonstrated how computing could transform business operations. Quantum computing is at a similar point today, searching for its equivalent – a game-changing application that validates its utility and drives widespread adoption.
Many believe that the first major use cases for quantum will emerge in areas like chemistry, where the technology’s unique strengths can be applied to molecular simulations, or in optimisation, where it could radically improve logistics, finance, and supply chain management. For AI, quantum’s efficiency offers the potential to handle the most computationally intensive tasks at a fraction of the current energy cost. As Chapman put it, “If you’re trying to hammer a nail, you need a hammer, not a banana.” Quantum computing, in this analogy, is the hammer – purpose-built for the job.
AI’s Power Consumption Problem and the Quantum Solution
The AI industry’s energy demands are only growing, driven by ever-larger models and the resources required to train them. Already, some tech companies are looking to nuclear energy to power their AI operations, a clear indication of the scale of the issue. But using quantum computing to handle high-demand AI tasks would allow companies to achieve better performance while drastically reducing energy consumption, offering a more sustainable alternative to expanding physical data centre infrastructure.
Beyond the environmental benefits, the cost savings alone make quantum a compelling option. When the necessary calculations can be run with a fraction of the energy, companies not only cut down on their operational costs but also free up resources to invest in further research and development, creating a positive feedback loop of innovation.
Toward a Sustainable Future for AI and Quantum
Quantum computing’s scalability is also critical for AI’s future. Every new generation of quantum hardware dramatically increases computational power due to the exponential scaling of qubits, whereas classical computing improvements are largely incremental. This rapid scaling means that within a few hardware cycles, quantum computing could handle tasks that would overwhelm even the largest classical data centres.
As early adopters in industries like pharmaceuticals and materials science begin to experiment with quantum-powered AI, the technology’s potential for mainstream adoption becomes clearer. Moving workloads from GPUs to quantum processors could make large-scale AI applications viable in fields where they were previously limited by cost and power constraints.
Final Thoughts: Quantum as the Right Tool for the Job
AI has reached a point where classical computing can no longer efficiently support its energy and processing needs. Relying solely on traditional GPUs and CPUs for tasks that require immense processing power is unsustainable. Quantum computing, with its ability to perform parallelised, probabilistic calculations, is the right tool for these challenges – allowing us to build advanced AI systems without an unsustainable rise in energy demands.
In short, as AI grows more complex, our computing solutions must evolve with it. Quantum computing stands as a promising path forward, poised to bring about the next leap in AI capability and efficiency. By embracing quantum, we’re not just looking for better performance; we’re choosing a future where AI can continue to expand sustainably.