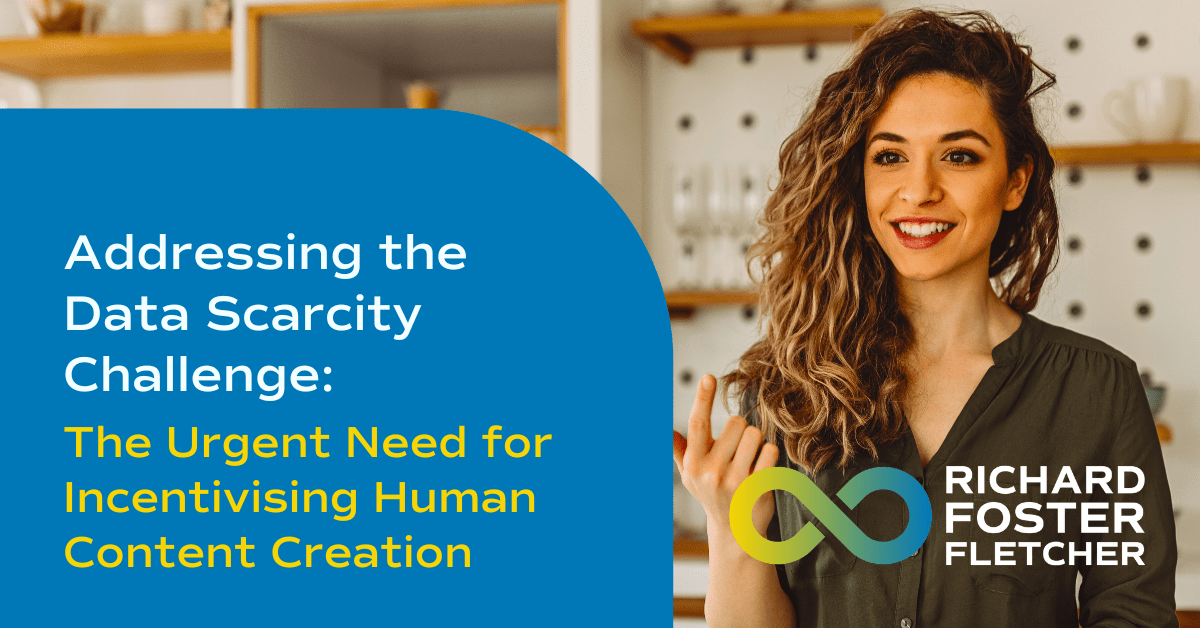
Addressing the Data Scarcity Challenge: The Urgent Need for Incentivising Human Content Creation

The availability of high-quality human-generated data is becoming a critical issue for training Large Language Models (LLMs). The fear that we might soon run out of this valuable resource is not unfounded and poses a significant threat to the development and effectiveness of AI systems. This challenge, if not addressed promptly, could lead to a scenario where AI models degrade in performance, producing low-quality outputs. This degradation is particularly problematic for businesses that rely on AI for decision-making, customer service, and competitive advantage.
The Magnitude of the Data Scarcity Problem
Human-generated content is essential for training AI models due to its richness and diversity. This data includes books, articles, scientific papers, and high-quality web content, all of which provide the varied and nuanced information that AI systems need to function effectively. As these sources become saturated or restricted, the influx of fresh, high-quality data diminishes, leading to potential degradation in AI performance.
Studies have shown that the accumulation of synthetic data, if used extensively, can lead to what researchers call “model collapse.” This phenomenon occurs when AI systems trained predominantly on AI-generated data lose their ability to produce accurate and reliable outputs (Nature) (ar5iv). The integrity of AI systems is thus heavily dependent on the continuous availability of high-quality human-generated data.
Why This Is a Business Issue
For businesses, the degradation of AI model performance due to data scarcity can have several adverse effects:
- Reduced Decision-Making Accuracy: AI-driven analytics and insights are crucial for informed decision-making in many businesses. Degraded AI models can lead to incorrect or suboptimal decisions, affecting strategic planning, resource allocation, and overall business performance. Inaccurate predictions and analyses can result in financial losses and missed opportunities.
- Compromised Customer Service: Many businesses use AI to enhance customer service through chatbots, personalised recommendations, and automated support systems. Poor AI performance can lead to unsatisfactory customer interactions, reduced customer satisfaction, and potential loss of clientele. Maintaining high-quality customer service is vital for retaining a competitive edge in the market.
- Increased Operational Costs: As AI model performance declines, businesses may need to invest more in manual interventions and corrections, leading to increased operational costs. Additionally, the cost of developing and maintaining AI systems could rise as more resources are needed to ensure data quality and model accuracy.
- Loss of Competitive Advantage: Companies that effectively leverage AI can gain significant competitive advantages through enhanced efficiency, innovation, and customer engagement. However, if AI models are compromised by low-quality data, businesses risk falling behind competitors who have better AI systems. This can lead to a decline in market position and profitability.
Urgent Solutions: Incentivising Content Creation
One of the most effective strategies to combat the issue of data scarcity is incentivising the creation of new, high-quality content. This approach can provide a continuous supply of valuable data for AI training, enhancing the performance and reliability of AI systems.
- Compensation for Creators: Financial incentives can significantly motivate individuals and organisations to produce high-quality content. By providing monetary rewards, we can ensure a steady stream of new data for AI training. This approach values the creators’ contributions and aligns their interests with the broader goal of sustainable AI development.
- Supportive Platforms: Developing and promoting platforms that support content creation can encourage more people to contribute. These platforms can offer tools, resources, and visibility for creators, fostering a community dedicated to generating high-quality data. A robust content creation ecosystem can ensure a continuous supply of diverse and valuable information.
- Recognition and Rewards: Recognising and rewarding high-quality content through awards, certifications, and public acknowledgement can serve as powerful motivators. This recognition not only benefits the creators but also ensures that the AI models are trained on the best possible data. Highlighting the importance of their contributions can drive creators to maintain high standards.
- Collaborative Initiatives: Partnerships between AI developers, content creators, and educational institutions can create a synergistic environment for content generation. Such collaborations can leverage the strengths of each party, resulting in innovative and high-quality content that benefits both AI training and the broader community. This collaborative approach can also help address specific data needs by guiding content creation efforts toward underserved areas.
Implementation: Who Pays and How?
Implementing these strategies requires careful planning and investment. Here are some potential approaches:
- Government Grants and Subsidies: Governments can play a crucial role by providing grants and subsidies to support content creation. By investing in the production of high-quality data, governments can ensure that AI systems remain robust and reliable, benefiting the broader economy and society.
- Corporate Sponsorships and Investments: Businesses that rely heavily on AI can sponsor content creation initiatives. By investing in the creation of high-quality data, companies can safeguard their AI systems’ performance and maintain a competitive edge. This approach aligns corporate interests with the goal of sustainable AI development.
- Public-Private Partnerships: Collaborations between public institutions and private companies can pool resources and expertise to support content creation. These partnerships can leverage government funding and corporate investment to create a sustainable pipeline of high-quality data.
- Crowdsourcing and Community Support: Platforms can be developed to crowdsource content creation, with community members contributing data in exchange for rewards or recognition. This approach taps into the collective effort of individuals and fosters a sense of ownership and collaboration.
The data scarcity challenge is a pressing issue that requires immediate attention. By incentivising human content creation through compensation, supportive platforms, recognition, and collaboration, we can address this problem effectively. This approach not only enhances the performance and reliability of AI systems but also aligns with the goal of fostering a thriving ecosystem for content creators. The urgency of this issue cannot be overstated, and proactive measures are essential to ensure that AI continues to evolve in a way that benefits society.