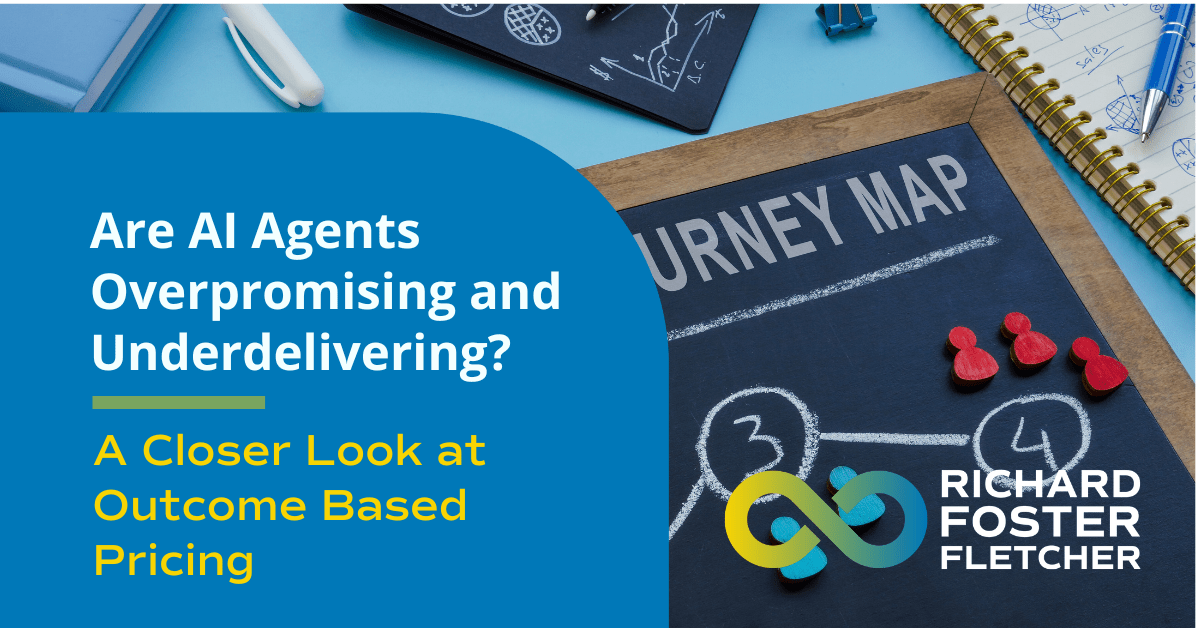
Are AI Agents Overpromising and Underdelivering? A Closer Look at Outcome-Based Pricing

AI agents have been framed as transformative tools, capable of reshaping industries and redefining workflows. Their proponents argue that technologies such as OpenAI’s ChatGPT agents or Salesforce’s Agentforce represent a new paradigm: tools that don’t just assist workers but potentially replace entire tasks, even entire roles. Central to these claims is the seductive idea of outcome-based pricing—a model where businesses only pay for results, such as tickets resolved, leads converted, or sales made.
It sounds ideal: a pricing structure that rewards success and mitigates the risk of adoption. But what if this isn’t a revolution but a sleight of hand? As the hype grows louder, it’s worth questioning the assumptions underpinning such models and the broader narratives around AI agents.
Is Outcome-Based Pricing a Real Innovation?
At its core, outcome-based pricing hinges on trust: trust in AI providers, trust in metrics, and trust in the systems used to assess success. Yet, the concept isn’t new. Similar approaches have existed in consulting, legal services, and advertising for decades. What’s different is its application to autonomous systems—machines that neither understand the tasks they perform nor are accountable for their outcomes.
This raises significant concerns. Unlike human service providers, AI systems operate as black boxes. Businesses relying on outcome-based pricing risk becoming locked into metrics that may not truly capture the value—or the risks—of these tools. For instance, a customer support AI measured solely on resolution rates could incentivise superficial responses, ignoring deeper customer satisfaction metrics.
Does this model reward real value, or does it encourage businesses to chase short-term gains at the expense of sustainable improvements?
The AI Economy: Efficiency or Exploitation?
The rise of AI agents coincides with an era of corporate focus on efficiency. Businesses face relentless pressure to cut costs and boost productivity, often at the expense of innovation and long-term vision. Outcome-based pricing fits neatly into this framework. It allows companies to frame AI adoption as a cost-neutral exercise while disguising the profound structural changes it entails.
But the model raises uncomfortable questions about labour, power, and agency. If AI is replacing human tasks, what happens to the displaced workers? Are businesses effectively offloading risk to technology, outsourcing blame to systems that cannot be held accountable?
Moreover, the rhetoric around outcome-based pricing often glosses over the inherent biases and limitations of AI systems. For example, who decides what counts as a “successful” outcome? And how do we ensure those definitions aren’t skewed in favour of the provider, especially when the metrics themselves are shaped by the very systems under evaluation?
Can AI Agents Deliver on Their Promises?
The bold claims of AI vendors—faster resolutions, higher conversions, seamless integrations—deserve scrutiny. Many AI systems are still in experimental stages, prone to errors and inconsistencies. Even in controlled environments, their performance is often less impressive than advertised.
Take the case of customer service AI, where “success” is measured by metrics such as resolution time or call deflection rates. These numbers may look good on paper, but they obscure the reality of poor customer experiences, unresolved issues, and frustrated employees tasked with cleaning up after the AI. Similarly, sales agents boasting higher conversion rates might rely on aggressive, impersonal tactics that alienate customers in the long run.
By focusing narrowly on quantifiable outcomes, businesses risk losing sight of broader goals: building trust, fostering loyalty, and creating meaningful connections. If AI agents can’t deliver on these less tangible—but no less important—measures of success, their promise may ultimately ring hollow.
Who Benefits from AI in the Workplace?
The push towards AI adoption often assumes a win-win scenario: businesses save money, employees are freed from mundane tasks, and customers enjoy better service. But this narrative ignores the potential downsides for those at the sharp end of the disruption.
Workers face the double burden of reduced autonomy and increased surveillance, as AI systems monitor and guide their every move. Customers encounter dehumanised interactions, stripped of the nuance and empathy that human agents provide. And businesses, lured by promises of cost savings, may find themselves locked into expensive, opaque systems that fail to deliver meaningful returns.
Outcome-based pricing may shift some of these risks away from businesses, but it doesn’t eliminate them. If anything, it raises the stakes, tying success to narrowly defined metrics that may not align with broader organisational goals.
The Bigger Questions
As AI agents proliferate, the conversation must go beyond technical capabilities and pricing models. What kind of economy are we building with these tools? Are we prioritising efficiency at the expense of resilience, adaptability, and human connection? And what happens when the outcomes we optimise for—speed, volume, cost—conflict with the values we claim to uphold?
The introduction of AI agents isn’t just a technological shift; it’s a cultural one. It challenges our assumptions about work, value, and accountability. It forces us to confront uncomfortable truths about power, inequality, and the trade-offs we’re willing to make in the name of progress.
The future of AI agents may well hinge on these broader questions. Will they be tools for empowerment, helping businesses and workers navigate an increasingly complex world? Or will they become symbols of an extractive economy, where value is reduced to metrics and human agency is sidelined in favour of automation?
For now, the promises of AI agents—like the outcome-based pricing models they enable—should be met with healthy scepticism. Not because they lack potential, but because their real impact will depend on the choices we make about how to use them. And those choices, more than any technology, will determine whether AI truly serves us—or simply replaces us.